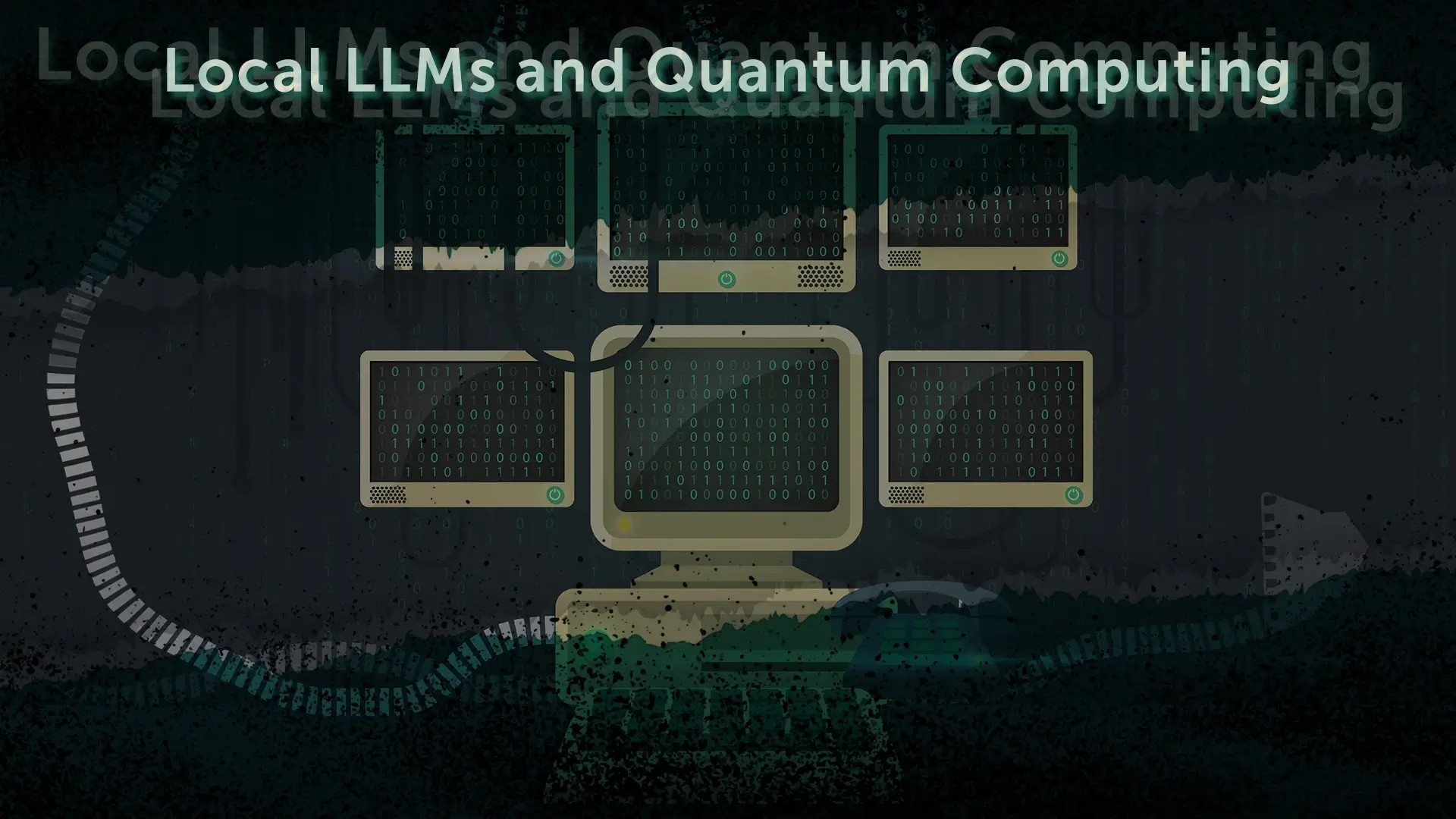
Image credit: Based on an image by macrovector / Freepik
Originally appeared in LinkedIn Future Singularity
Have you ever considered the possibility of running advanced AI models on your personal computer? I’m not talking about just simple neural networks, but complete language models capable of performing complex analysis and generation tasks. As technology advances, a future where this is possible may be closer than we think, especially given the parallel development of quantum computing.
The Current State of AI Technology
We should really take a step back. Before we can look that far forward, we have to first understand where we are now. Modern Large Language Models (LLMs) can typically have hundreds of billions of parameters and demand significant computer resources for training the models and inference. Models such as GPT-4 and Claude are believed to train on hundreds of high-end GPUs, requiring the energy equivalent to that of small towns.
However, recent advances in something known as model optimization have led to encouraging outcomes. Quantization, pruning, and knowledge distillation have already decreased model sizes by a factor of ten or more while retaining much of their functionality. Companies like Meta have successfully developed tiny models that can run on mobile devices, albeit with less functionality than their larger counterparts.
The Convergence of AI and Quantum Computing
Currently, if you want to run some of the popular and powerful AI models, it will require substantial computational resources – the kind that are typically only found in large data centers with immense power requirements. These models also demand considerable processing power and memory to handle their billions of parameters I mentioned before.
However, the efficiency of AI technology is improving at a somewhat remarkable pace. For instance, operations that once required hundreds of servers can now be performed on a few specialized GPUs. Researchers are making significant strides in model compression and optimization, which allows these systems to accomplish more while using fewer computational resources.
A Potential Game-Changer
Quantum computing adds a completely new paradigm to this equation. In contrast to classical computers, which process information in bits (1s and 0s), quantum computers use qubits, which can exist in multiple states at the same time due to quantum mechanics. This fundamental difference has the potential to revolutionize how we handle AI workloads.
Understanding Quantum Advantages
Current quantum computers are still in their early stages, with the most advanced systems containing approximately 1000 qubits. However, their potential for certain types of calculations is enormous. In the context of AI applications, quantum computing has transformative potential. The ability to efficiently process complex probability distributions could change the way neural networks are trained. When we consider the effort involved in training or parameter optimization, a critical aspect of AI model development, quantum algorithms designed specifically for this purpose have the potential to see dramatic improvements.
The ability of quantum systems to analyze large amounts of data at once may improve pattern recognition capabilities. This could lead to more advanced AI models that can detect subtle patterns and relationships that current systems may overlook. The quantum advantage in handling complex mathematical operations may also open up new approaches to knowledge representation and processing that are not possible with classical computing.
The Path to Personal AI Infrastructure
The development of personal AI systems will involve the intricate interaction of numerous technological advancements rather than merely considerations around raw processing power. Model efficiency improvements are driving innovation in architecture design, resulting in systems with fewer parameters while still providing high performance. These advancements are poised to allow for augmentation by innovative approaches to knowledge representation and storage, which can make models more compact and efficient.
Hardware advancements are equally important in this evolution. Consider new chip designs that are emerging which are specifically tailored to AI workloads, providing improved performance while using less power. Memory architectures are being redesigned to better meet the specific needs of AI operations, and energy efficiency is improving across the board.
The software ecosystem that underpins these developments is also maturing rapidly. Model deployment and management tools are increasingly sophisticated and user-friendly. Security frameworks are evolving to protect local AI systems from potential threats, and data handling methods are becoming more efficient and dependable.
Technical Challenges and Considerations
The path to personal AI systems still faces several significant technical challenges. Heat management presents a unique challenge because intensive processing generates significant thermal output that must be effectively managed. Power consumption remains a major concern, particularly for personal devices that must operate within reasonable energy limits.
Memory bandwidth constraints may limit performance, while storage requirements for model weights and data present their own challenges. Security considerations are equally important; protecting against model manipulation and ensuring data privacy are critical issues that require strong solutions.
Societal Implications
The widespread availability of powerful local AI systems may result in far-reaching consequences for society. The job market would likely undergo significant transformation, with new roles emerging for the management and maintenance of personal AI systems. Traditional jobs could evolve to include AI assistance, while completely new career paths could emerge.
These changes would necessitate an adaptation in the educational landscape. The technical curriculum would need to be updated to include AI system management and maintenance. As artificial intelligence systems become more common in everyday life, public awareness and digital literacy programs will become equally and increasingly important.
Ethical concerns would likely shoot to the forefront. As AI systems become more personalized and localized, concerns about responsible use, fairness, and accountability become more pressing and will likely result in greater scrutiny. Organizations and individuals would have to make complex decisions about AI deployment and usage while also considering some of these broader societal implications.
Looking Forward
The future of personal AI systems, aided by quantum computing capabilities, appears to be an extremely exciting opportunity, but at the same time an extraordinarily complex challenge for the technology industry. Success will require a careful balance of technological advancement with practical considerations and a grounding of ethical obligations.
As these technologies advance, maintaining a realistic perspective while preparing for transformative changes is key. The future of personal AI systems may be closer than we realize, but it will most likely come gradually, with each advancement building on previous successes and learning from previous challenges.
The transition from cloud-based services to locally hosted AI systems could be a watershed moment in computing history, similar to the switch from mainframes to personal computers. This transformation will necessitate ongoing innovation in hardware, software, and system design, as well as careful consideration of the societal consequences.
*Note: This post is based on current technological trends and theoretical developments. This blog post was written by a human who still occasionally forgets where they put their keys, so take all future predictions with a grain of salt. Actual timelines and capabilities may vary as these technologies continue to evolve.